Generative AI for Synthetic Data
Generative AI is currently in the spotlight with the release of ChatGPT, but it has already been making significant contributions to data and analytics (D&A) through synthetic data. This solution can help fill gaps in real-world data sources and even improve model outcomes. How are data and analytics professionals currently using synthetic data and what challenges do they face?
One minute insights:
Organizations adopt AI-generated synthetic data because of challenges with real-world data accessibility, complexity and availability
Partially synthetic data is the most common approach and text-based is the most-used type of synthetic data
Leaders have seen improvements in model accuracy and efficiency as a result of synthetic data
Most challenges with synthetic data are inherited from limited, poor quality or biased real-world source data
To ensure synthetic data quality, most leaders have implemented best practices like using multiple data sources and synthetic dataset validation
Challenges with real-world data accessibility, complexity and availability have led organizations to adopt AI-generated synthetic data
Most IT and D&A leaders surveyed say their organization adopted AI-generated synthetic data because of challenges with real-world data accessibility (60%), complexity (57%), or availability (51%).
3% of respondents say their organization did not face any challenges with real-world data.
Which challenges has your organization faced with real-world data that led to adopting AI-generated synthetic data? Select all that apply.
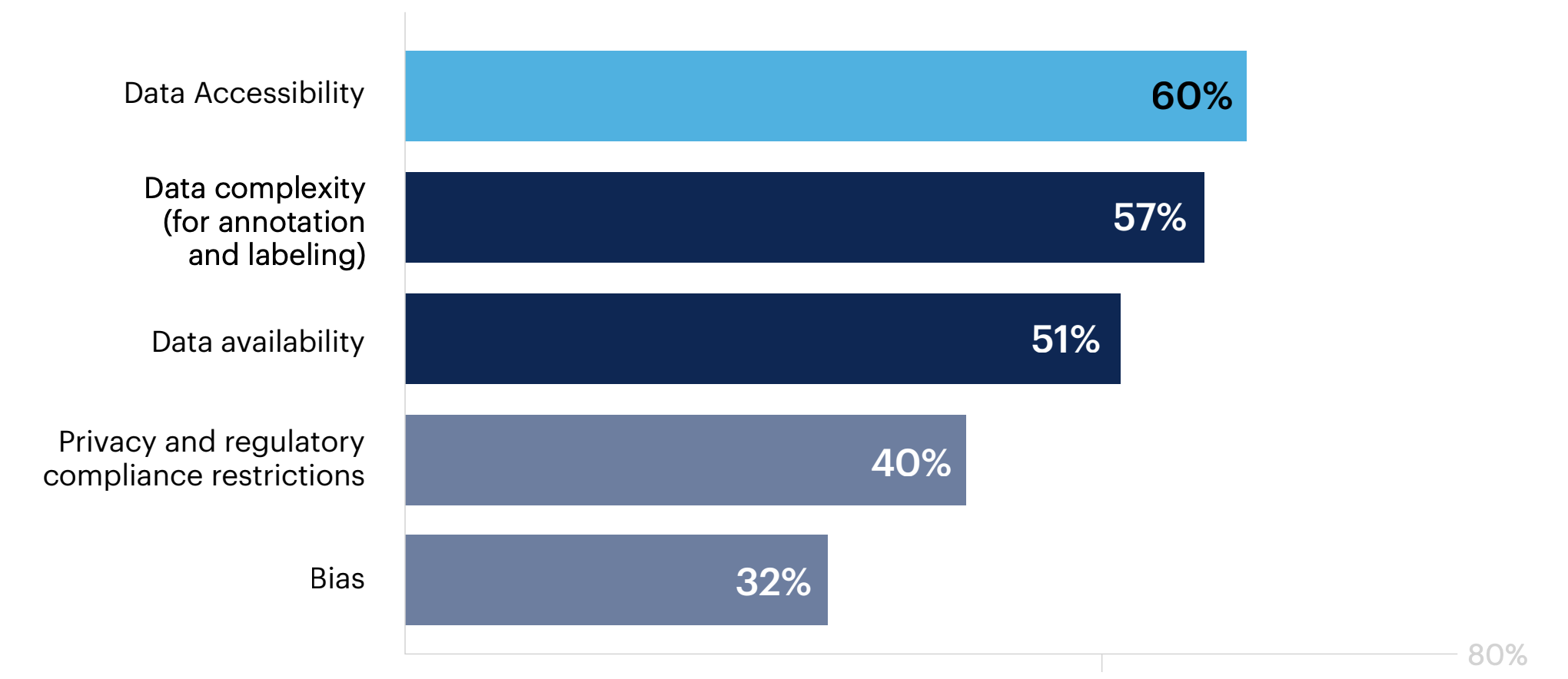
n = 150
Unbalanced data 31% | We haven’t faced any challenges with real-world data 3% | Other 0%
Thoughts on using and creating AI-generated synthetic data
Question: Do you have any final thoughts to share on AI-generated synthetic data?
Models have to be continuously trained and synthetic data is helping us very much.
This is one area where AI can really help.
Fully synthetic data is less likely to be used than partially synthetic data; text-based is the most common type
Most respondents say their organization uses partially synthetic data (63%) or a combination of partially and fully synthetic data (20%).
Does your organization use fully or partially synthetic data?
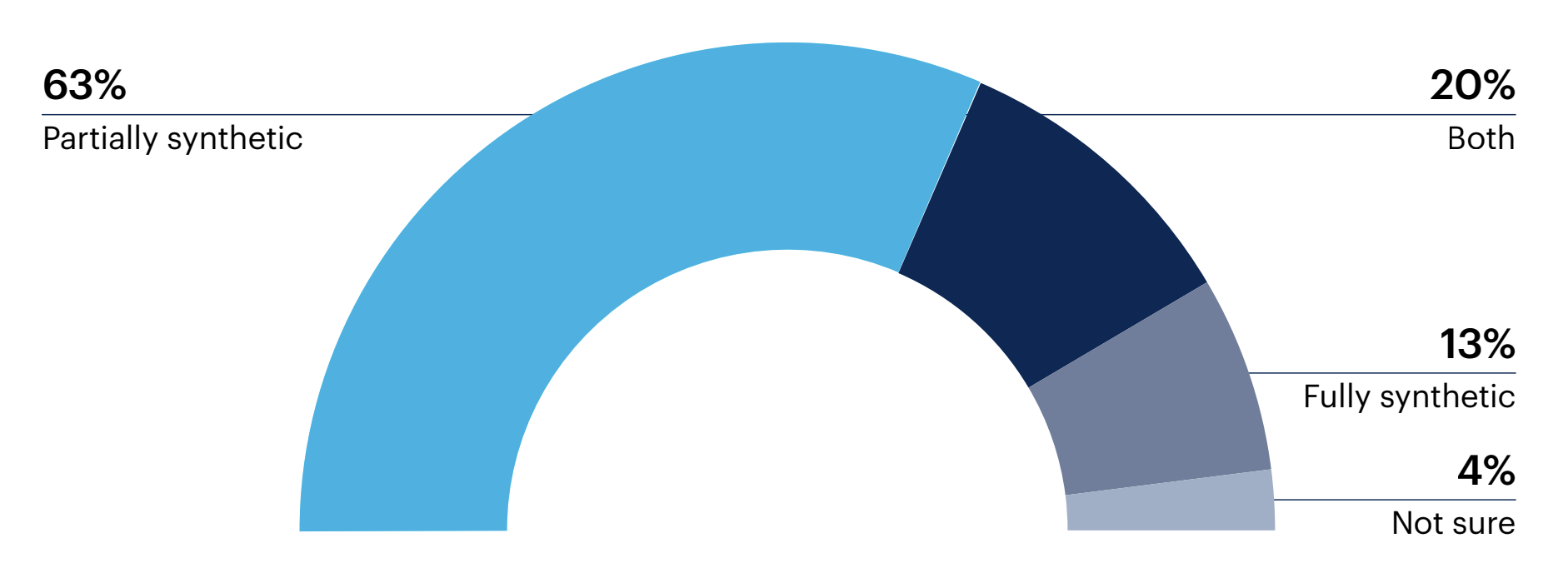
n = 150
As for the types of synthetic data, text-based is used by an overwhelming majority of respondent organizations (84%). Image-based (54%) and tabular (53%) synthetic data are each used at more than half of respondent organizations.
What type(s) of synthetic data are being used in your organization? Select all that apply.
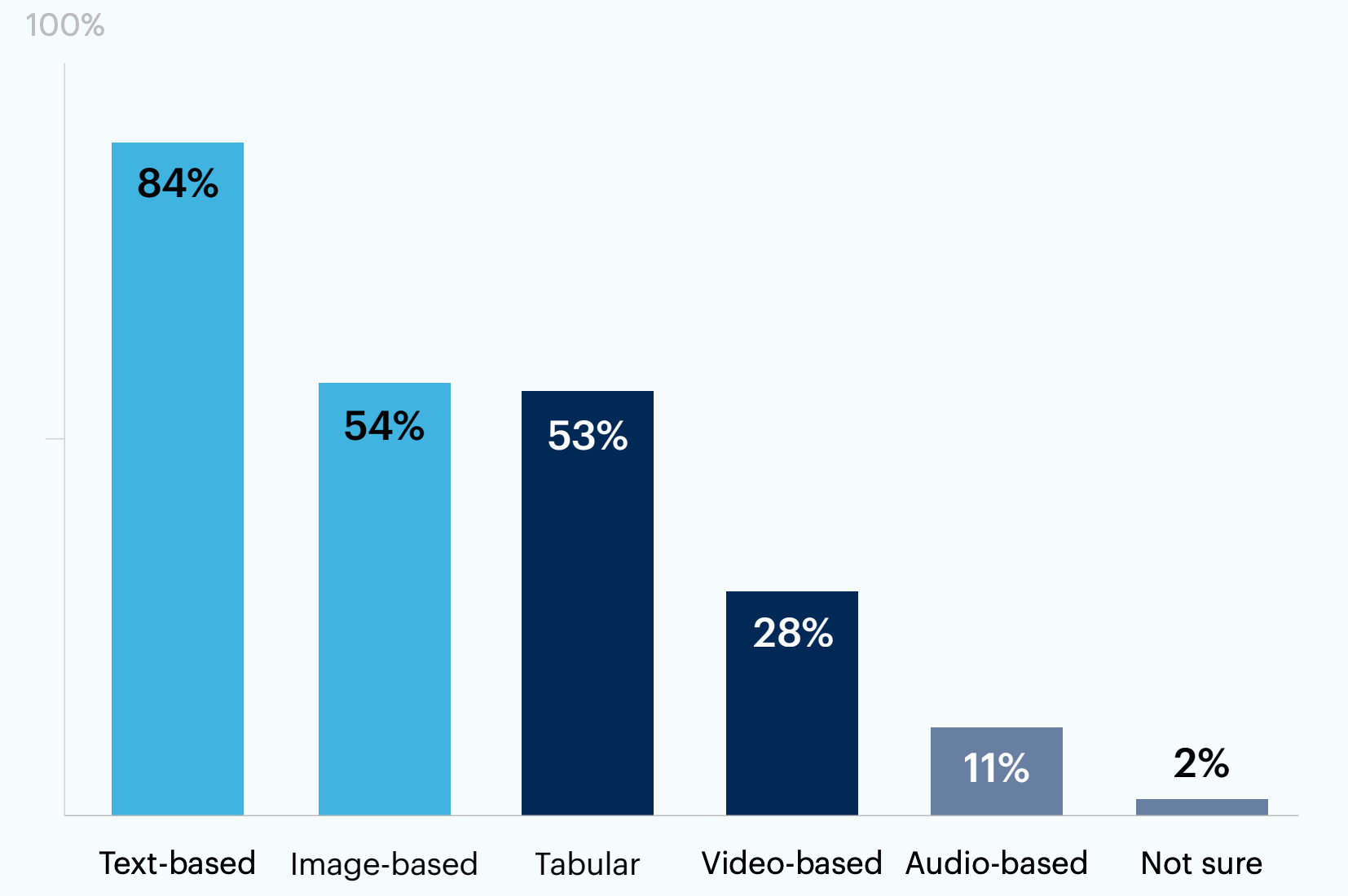
n = 150
50% of respondents say their organization generates synthetic data through a custom-built solution with open-source tools, while 31% turn to vendor solutions to generate their synthetic data.
How is synthetic data generated at your organization?
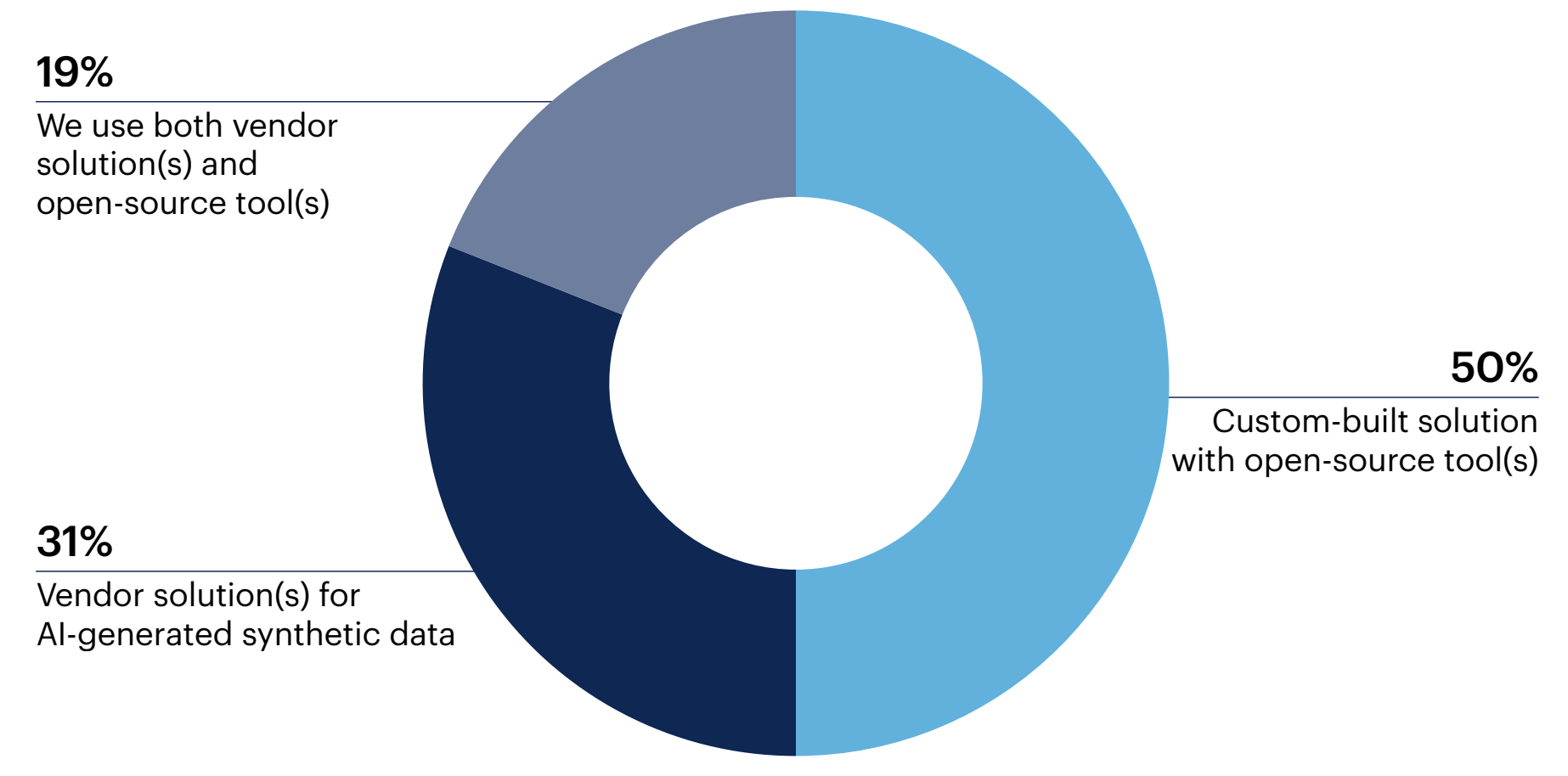
n = 150
Other 0%
Concerns and challenges with AI-generated synthetic data
Question: Do you have any final thoughts to share on AI-generated synthetic data?
It is in [an] early stage and will be tough to adopt across [the] entire organization and also ROI cannot be [easily] calculated. Regulatory issues are a major concern.
AI generated [techniques have] a high level of myopic bias, selecting the right vendor for data remains a challenge.
Synthetic data can improve model accuracy and eiciency, but many have faced challenges with lack of or low quality real-world source data
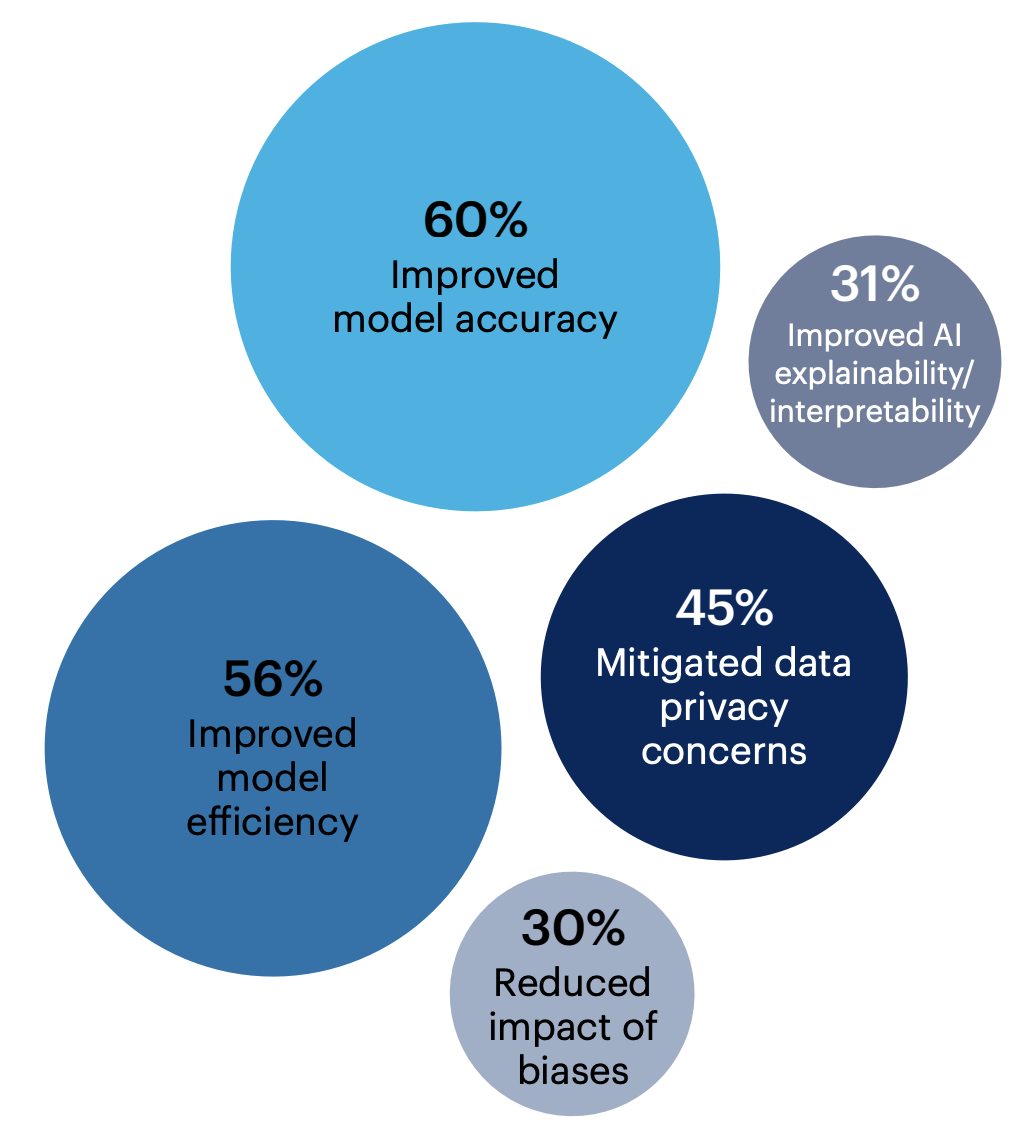
The most often realized benefits of synthetic data at respondents’ organizations are improved model accuracy (60%), improved model efficiency (56%) and mitigated data privacy concerns (45%).
How has synthetic data benefited your organization? Select all that apply.
Increased efficiency of data teams 25% | Rebalanced datasets 23% | Reduced data breach risks 19% | Reduced overfitting 14% | None of these 3% | Other 0%
n = 150
About half (51%) of respondents have dealt with a lack of real-world source data for the synthetic data at their organization. More than one-third have experienced challenges with inherited bias in synthetic data (46%), low quality real-world source data (41%) or inaccuracy caused by statistical noise (34%).
Only 2% of respondents have not experienced any challenges with synthetic data at their organization.
What challenges have you experienced with synthetic data in your organization? Select all that apply.
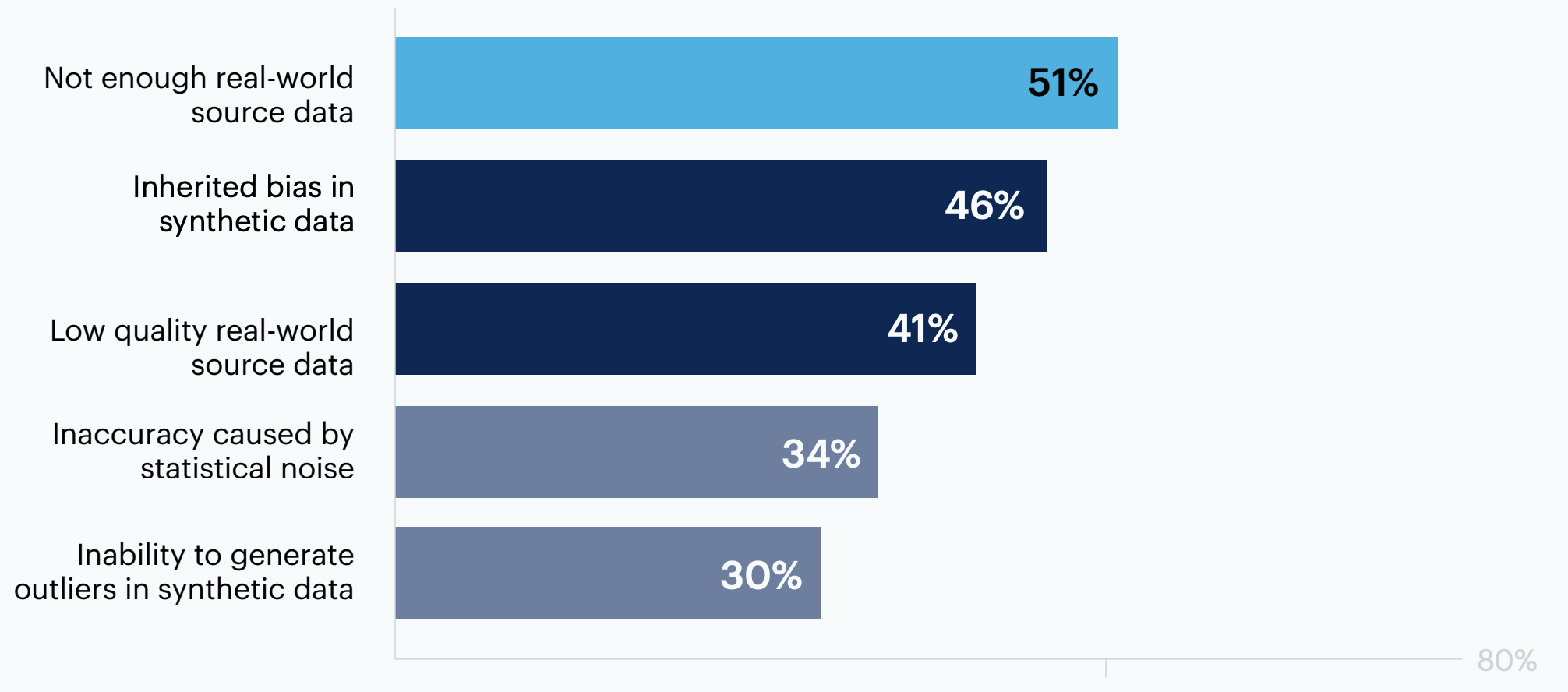
n = 150
Lack of expertise 25% | Insufficient resources 24% | Accuracy degradation 23% | Integration with existing data systems 14% | Cost of computing power 12% | Selecting the right vendor 11% | Determining appropriate utility metrics 11% | Legal/ethical concerns (e.g., re-identification risk) 10% | We haven’t experienced any challenges with synthetic data 2% | Other 0%
Most have implemented best practices to ensure quality of their synthetic data
65% of respondents use multiple data sources for generative models to ensure their synthetic data quality is high. Synthetic dataset validation (59%) and data quality checks before use in generative models (50%) are also common best practices among respondents.
What best practices have you implemented to ensure the quality of your synthetic data is high? Select all that apply.
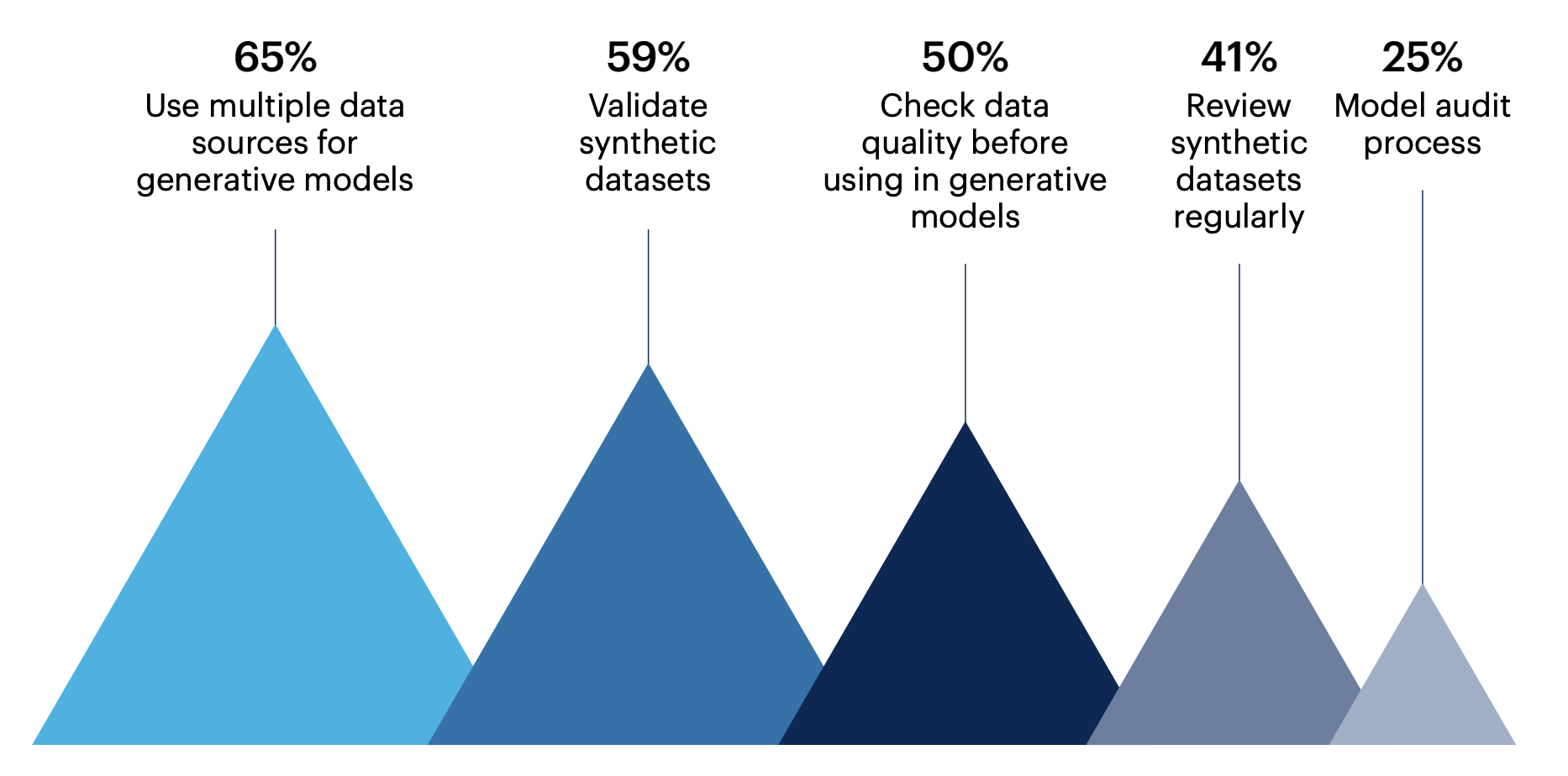
n = 150
Evaluate synthetic data quality 24% | We haven’t implemented any best practices 7% | Other 0%
Risks and considerations for AI-generated synthetic data
Question: Do you have any final thoughts to share on AI-generated synthetic data?
AI generated synthetic data is quite sensitive and needs to be handled securely.
AI-generated synthetic data has potential benefits, but ethical considerations and limitations in accuracy and usefulness must be considered.
There has to be [an] integration of Human Resource insights along with AI generated synthetic data to improve the utmost effectiveness.

Want more insights like this from leaders like yourself?
Click here to explore the revamped, retooled and reimagined Gartner Peer Community. You'll get access to synthesized insights and engaging discussions from a community of your peers.
Respondent Breakdown
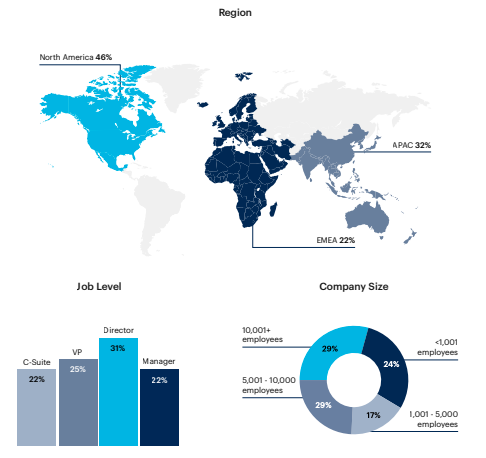
Note: May not add to 100% due to rounding Respondents: 150 IT and D&A leaders who work with or oversee groups that work with AI-generated synthetic data at their organization